Researchers at Houston Methodist Research Institute have constructed an artificial intelligence very adept at detecting breast cancer.
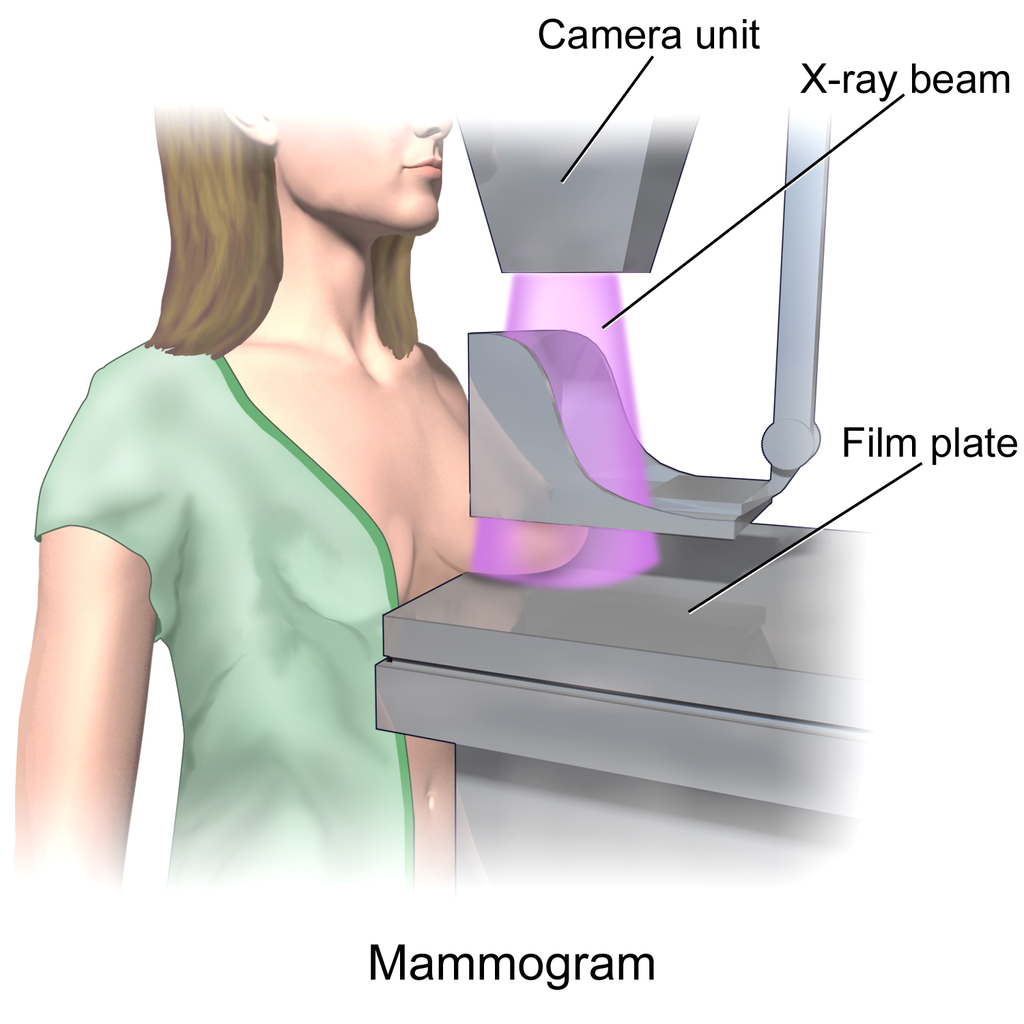
Lead researcher Stephen Wong told Science Daily that the software is designed to continually get smarter and that it is able to process millions of patient records within a very short time.
This processing power enables the artificial intelligence to compare data in a huge database of both research and previous cancer samples to better determine whether women’s mammograms are cancer or at risk of cancer.
By analyzing and cross-examine doctors’ medical notes, research, and patient’s personal medical history, the software can now diagnose breast cancer with 99 percent accuracy. It is reportedly 20 times more accurate than a human doctor.
Stephen T. Wong, Ph.D., is chair of the Department of Systems Medicine and Bioengineering at Houston Methodist Research Institute. Jenny C. Chang, M.D., director of the Houston Methodist Cancer Center used the AI software to evaluate mammograms and pathology reports of 500 breast cancer patients.
The artificial intelligence identified all specific subsets of cancer for all woman within a few hours – 30 times faster than a doctor would.
Science Daily reports that manual review of 50 charts took two clinicians 50-70 hours. The AI reviewed 500 charts in a few hours, saving over 500 physician hours.
Mammography is a widely used method to detect breast cancer in many countries. But the method has its shortcomings. Every other woman are diagnosed with breast cancer in the US.
This new technology would mitigate the large number of women who are called to follow-up biopsies, of which many are unnecessary. Over 1.6 million breast biopsies are performed annually of which 20 percent are unnecessarily performed due to false-positive mammogram results of cancer-free breasts, according to the American Cancer Society.
The research team now hopes this artificial intelligence software will help physicians better define the percent risk requiring a biopsy, increasing the precision and equipping doctors with a tool to decrease unnecessary breast biopsies.
Reference:
Tejal A. Patel MD, Mamta Puppala MS, Richard O. Ogunti MBBS, Joe E. Ensor Ph.D., et. al. Correlating mammographic and pathologic findings in clinical decision support using natural language processing and data mining methods